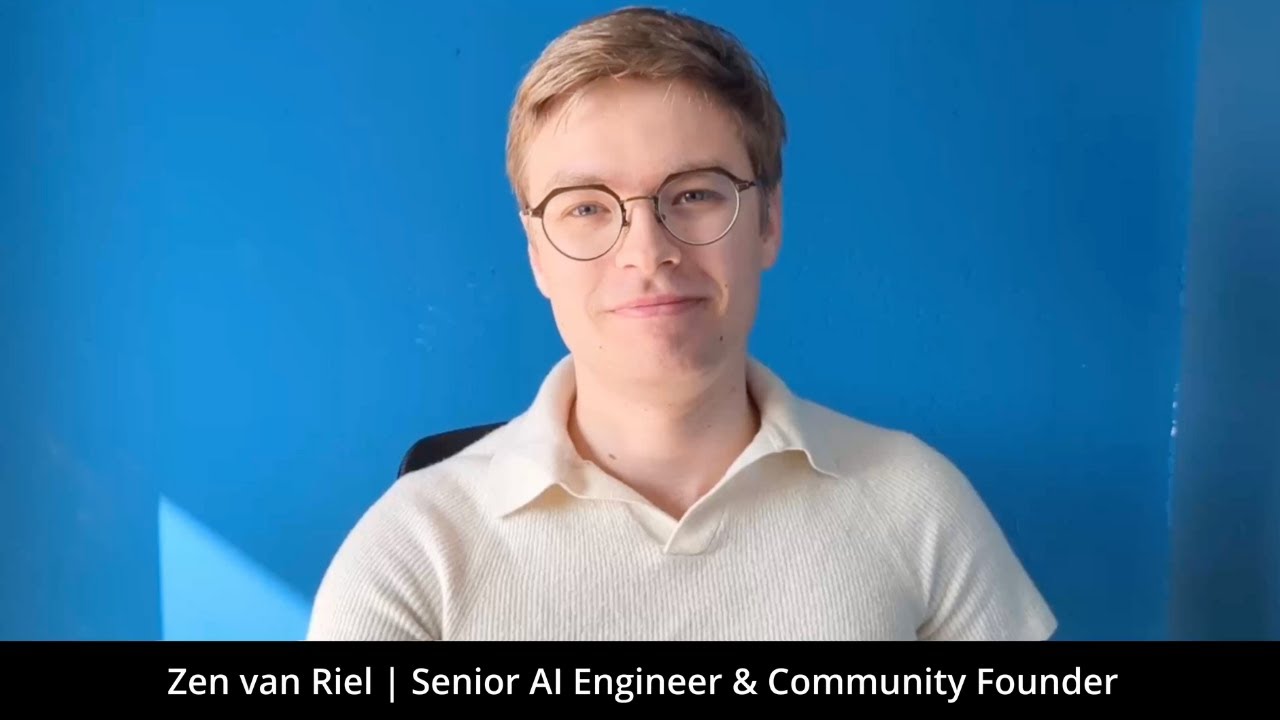
Building Your Career in AI
When I look back at my own journey from a passionate self-taught programmer to a senior engineer at a big tech company, one truth stands out clearly: the most reliable AI career path focuses on implementation engineering rather than research or theory. I learned this firsthand as I condensed what would typically be a decade-long career into just four years. While academia emphasizes theoretical knowledge, companies urgently need professionals who can build reliable, production-ready systems that deliver real business value.
Implementation vs. Research Paths
AI career paths broadly fall into two categories:
- Research-focused: Advancing models through theoretical innovation
- Implementation-focused: Building working systems using existing models
While both have value, implementation engineering offers greater job availability, faster entry, and often comparable compensation without advanced degrees.
Career Progression Timeline
The implementation-focused AI career typically progresses through:
- Entry implementation role (6-12 months): Basic AI system building
- Mid-level implementation (1-3 years): Creating reliable production systems
- Senior implementation (3+ years): Architecting complex AI solutions
- Lead/Principal roles (5+ years): Directing technical strategy for AI systems
This progression can occur significantly faster than research-focused paths. In my case, I went from being a self-taught developer to working at a major tech company within just a year, and then quickly progressed to senior roles by focusing relentlessly on implementation skills rather than theoretical credentials.
Compensation Advantages
Implementation-focused AI engineers often achieve:
- Entry salaries of $80,000-$120,000
- Mid-level compensation of $120,000-$180,000
- Senior compensation of $180,000-$250,000+
- Additional opportunities through specialized consulting
These figures compare favorably to research paths while requiring less initial credentialing. I’ve experienced this compensation trajectory firsthand, nearly tripling my income since starting as a new graduate by focusing on solving real business problems with modern AI implementation skills.
Fastest Path to Career Success
The most direct route into this career path focuses on:
- Building complete systems rather than understanding every model detail
- Developing deployment and monitoring expertise
- Learning cloud infrastructure for AI workloads
- Creating a portfolio of implementation projects
This practical approach addresses what employers actually need from AI professionals. In my own toolkit, these skills have been foundational – from understanding tokens, embeddings and vectors to mastering cloud AI models and practical implementation techniques like retrieval augmented generation. What’s mattered most in my career hasn’t been theoretical knowledge about how models work internally, but the ability to bring AI solutions from proof of concept all the way to production.
Ready to pursue an implementation-focused AI career path with clear progression? Join the AI Engineering community where I’ll share the exact roadmap I used to build production AI systems at scale. You’ll get structured learning designed by practitioners who’ve successfully navigated this career trajectory, with guidance tailored to developing the marketable implementation skills that actually matter in today’s AI job market.