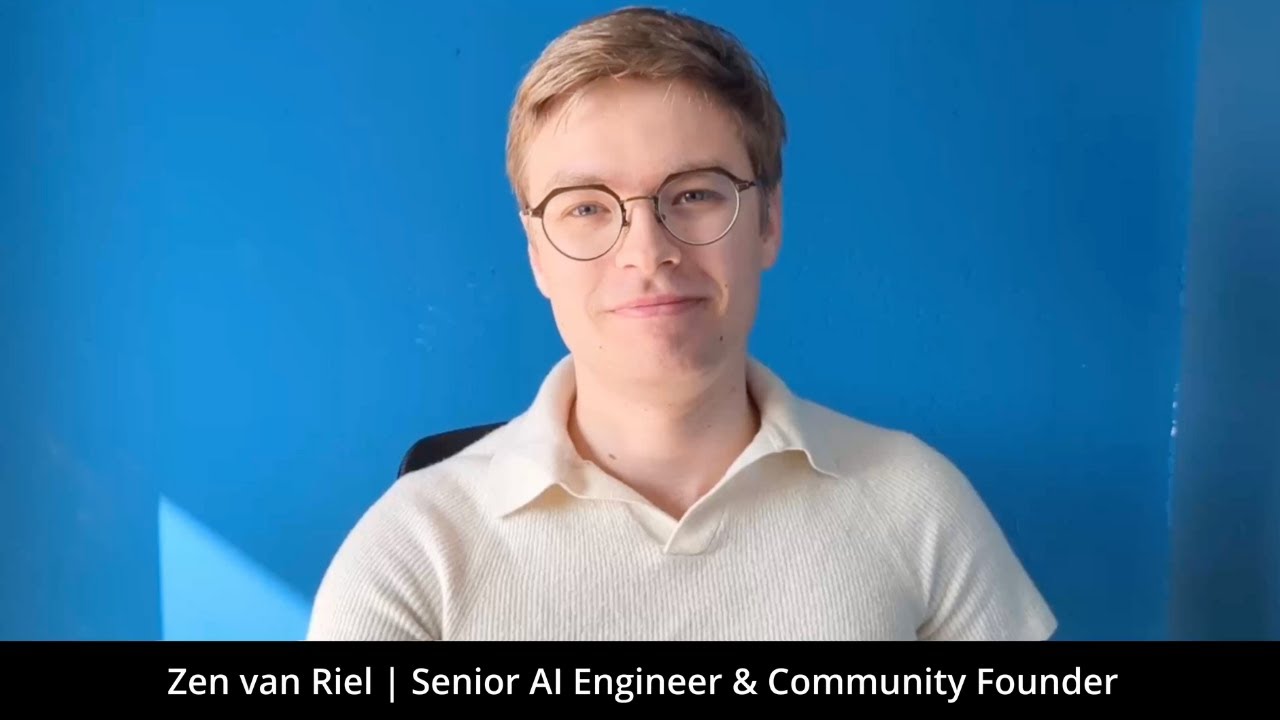
The Implementation Skills In Demand for AI Engineering Jobs
While AI job listings often emphasize theoretical knowledge and credentials, hiring managers consistently report that implementation skills are their most urgent need. Understanding this disconnect creates a strategic advantage for job seekers focusing on practical capabilities.
The Implementation Skills Gap
Companies implementing AI face a critical shortage of engineers who can:
- Design complete systems that integrate AI components effectively
- Deploy reliable solutions that operate at scale
- Manage costs while delivering appropriate performance
- Create maintainable architectures that other engineers can extend
This gap exists despite a surplus of candidates with theoretical AI knowledge.
Most-Requested Implementation Skills
Current AI engineering job descriptions increasingly emphasize:
- Experience building complete AI systems from concept to production
- Familiarity with deployment infrastructure and monitoring
- Understanding of data processing pipelines and vector storage
- Knowledge of integration patterns with existing systems
These practical capabilities often matter more than deep mathematical understanding.
Beyond Technical Implementation
The most in-demand engineers combine implementation with:
- Business context understanding and problem definition
- Ability to communicate AI capabilities to non-technical stakeholders
- Pragmatic approach to system design and resource constraints
- Experience handling real-world data limitations
This broader skillset addresses challenges companies actually face when deploying AI.
The Experience Paradox Solution
Communities offer a solution to the classic experience paradox—needing experience to get experience:
- Building production-grade projects with proper guidance
- Solving real implementation challenges in collaborative environments
- Receiving feedback from experienced practitioners
- Creating portfolio work that demonstrates practical capabilities
These activities develop and demonstrate the skills employers actually need.
Want to develop the implementation skills employers are desperately seeking? Join the AI Engineering community for structured learning pathways focused on building complete AI systems, guided by practitioners who understand what companies actually need from AI engineers.