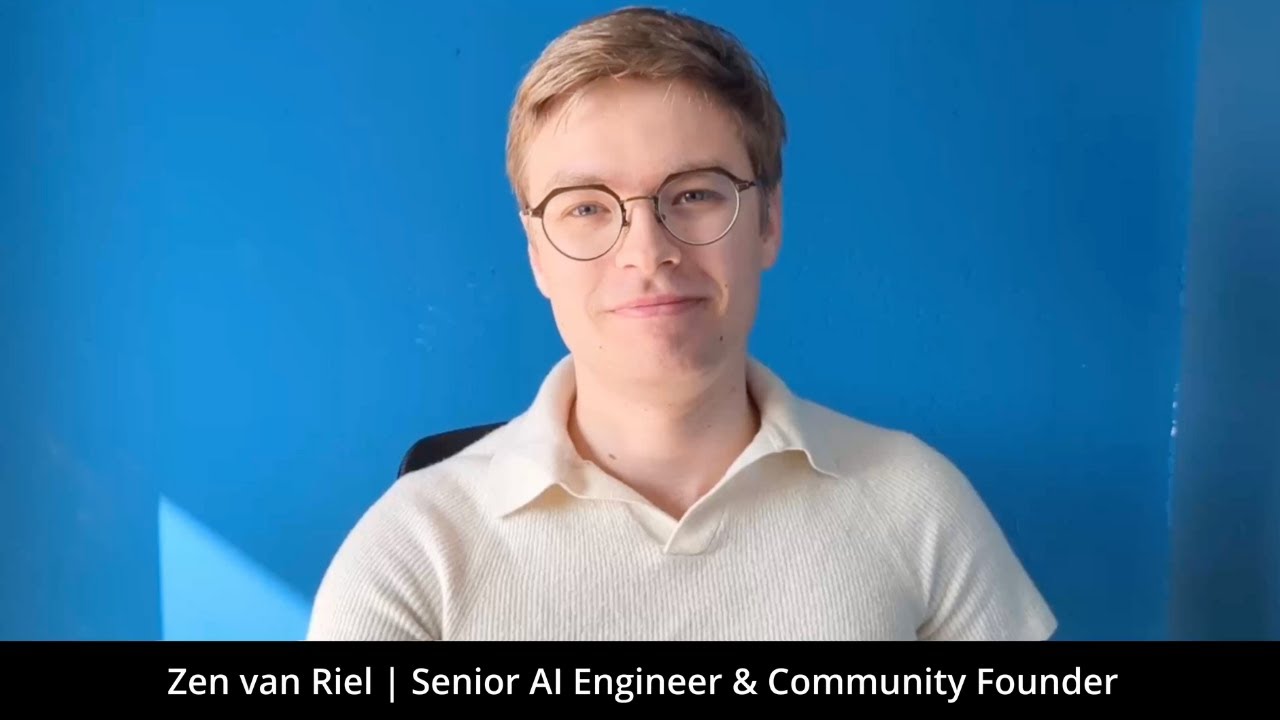
AI Project ROI
As organizations increasingly invest in artificial intelligence, the ability to measure and communicate the return on these investments becomes critical. Yet traditional ROI calculations often fall short when applied to AI initiatives, leading to either unrealistic expectations or an inability to demonstrate actual value. Throughout my experience implementing AI solutions at scale, I’ve found that successful ROI assessment requires approaches specifically adapted to the unique characteristics of AI projects.
Why Traditional ROI Calculations Fail for AI Projects
Standard ROI approaches often prove inadequate for AI initiatives because they don’t account for how AI creates value differently from traditional technology investments. AI projects frequently deliver cumulative benefits that grow over time rather than immediate returns, making traditional time-bound ROI calculations misleading.
Many AI benefits manifest through improved decision quality rather than direct cost reduction, creating attribution challenges when trying to isolate the specific contribution of AI within larger business processes. AI often generates unexpected benefits beyond the initial project scope – capabilities that weren’t even considered in original business cases but end up delivering substantial value.
Perhaps most significantly, traditional ROI typically ignores organizational learning and capability building, treating each project as an isolated investment rather than part of a broader AI maturity journey. Addressing these challenges requires ROI approaches specifically designed for the unique characteristics of AI implementations.
Practical Frameworks for AI ROI Calculation
Rather than relying on generic financial formulas, effective AI ROI assessment applies structured frameworks tailored to different project types. For AI projects that enhance existing business processes, the Process Efficiency Model works well. Start by documenting current process costs, time requirements, and quality metrics to establish a clear baseline. Then measure specific improvements in speed, resource utilization, and error rates after implementation. Convert these operational improvements into financial terms – calculating labor cost savings, capacity gains, and error reduction impacts. Account for the improvement trajectory as the system matures and factor in realistic implementation timelines rather than assuming immediate perfect utilization.
When AI projects improve decision quality rather than operational efficiency, the Decision Enhancement Framework is more appropriate. Begin by estimating the financial impact of current decision processes – including both the cost of making decisions and the results they generate. Quantify how AI enhances decision accuracy, consistency, or speed, and track actual results against historical performance baselines. Where possible, implement counterfactual analysis by comparing AI-assisted decisions with traditional approaches on identical scenarios. Finally, connect improved decisions to business outcomes like revenue, retention, or risk reduction.
For AI that primarily enhances customer interactions, the Customer Experience Valuation approach works best. Identify specific customer touchpoints affected by implementation and connect experience improvements to established satisfaction metrics. Measure changes in conversion, retention, and purchasing behavior, then translate these behavioral changes into customer lifetime value impact. The final step is estimating value across the customer base based on adoption patterns.
Practical ROI Measurement Approaches
Beyond these frameworks, specific measurement techniques can significantly improve AI ROI assessment accuracy and credibility. Controlled experimentation provides the most reliable evidence by deploying AI capabilities to a subset of processes or customers while maintaining control groups using traditional approaches. By comparing outcomes using consistent metrics, you can directly measure impact without confusion from other variables.
When experimental controls aren’t practical but reliable historical data exists, progressive baseline comparison works well. Document comprehensive performance metrics before implementation, then measure the same metrics at regular intervals after deployment. Adjust for other factors that might influence performance, calculate improvement rates, and project long-term impact based on observed trajectories.
For situations where direct measurement presents challenges, connect AI capabilities to established business value metrics through proxy metric correlation. Identify existing KPIs with clear financial value connections, establish how AI capabilities influence these metrics, and measure the correlation between AI utilization and KPI changes.
Accounting for Full AI Project Costs
Accurate ROI requires comprehensive cost accounting beyond initial development. Many organizations significantly underestimate implementation costs by focusing exclusively on model development while overlooking integration with existing systems, process redesign, interface development, testing across varied scenarios, and documentation creation. These costs frequently exceed the initial AI model development investment.
Operational considerations further impact ROI calculations. Account for ongoing expenses including cloud computing or infrastructure costs, API or service fees, data storage and processing requirements, monitoring and maintenance staffing, and regular evaluation and retraining cycles.
Perhaps most overlooked are organizational change factors – training for users and administrators, process change management, temporary productivity impacts during transition, governance requirements, and ongoing user support. These human and organizational factors often determine whether technical capabilities deliver actual value or become expensive shelf-ware.
Common AI ROI Pitfalls and How to Avoid Them
Several common mistakes consistently undermine effective AI ROI assessment. The “full automation fallacy” occurs when calculations assume complete replacement of human activities, despite the reality that most AI implementations augment rather than replace humans. Calculate value based on human-AI collaboration scenarios instead, tracking how AI changes human productivity and effectiveness while considering quality improvements beyond cost reduction.
Another frequent error is capability maturity oversight, where ROI projections assume full capability from initial deployment. In reality, AI systems typically improve substantially after implementation as they process more data and receive feedback. Model a maturity curve for system performance, track improvement rates, and extend ROI timelines to capture improvement-driven value.
Many organizations fall victim to indirect value exclusion by focusing solely on direct cost savings while overlooking how AI creates substantial value through indirect improvements. Identify and measure secondary benefits, connect AI capabilities to broader business outcomes, and capture delayed benefits that develop over extended periods. This comprehensive view prevents undervaluing AI investments that deliver multifaceted returns.
Conclusion: Practical Value Measurement for AI Success
Effective AI ROI assessment requires frameworks and approaches specifically adapted to the unique characteristics of these investments. By applying structured measurement techniques, accounting for full implementation costs, and avoiding common pitfalls, organizations can develop realistic expectations for AI initiatives while demonstrating their genuine business impact.
Rather than forcing AI projects into traditional ROI formulas, develop measurement approaches that capture their distinctive value creation patterns, including progressive improvement, decision enhancement, and capability building. This practical approach to ROI creates accountability for AI investments while recognizing their unique contribution to business success.
If you’re interested in learning more about AI engineering, join the AI Engineering community where we share insights, resources, and support for your journey. Turn AI from a threat into your biggest career advantage!