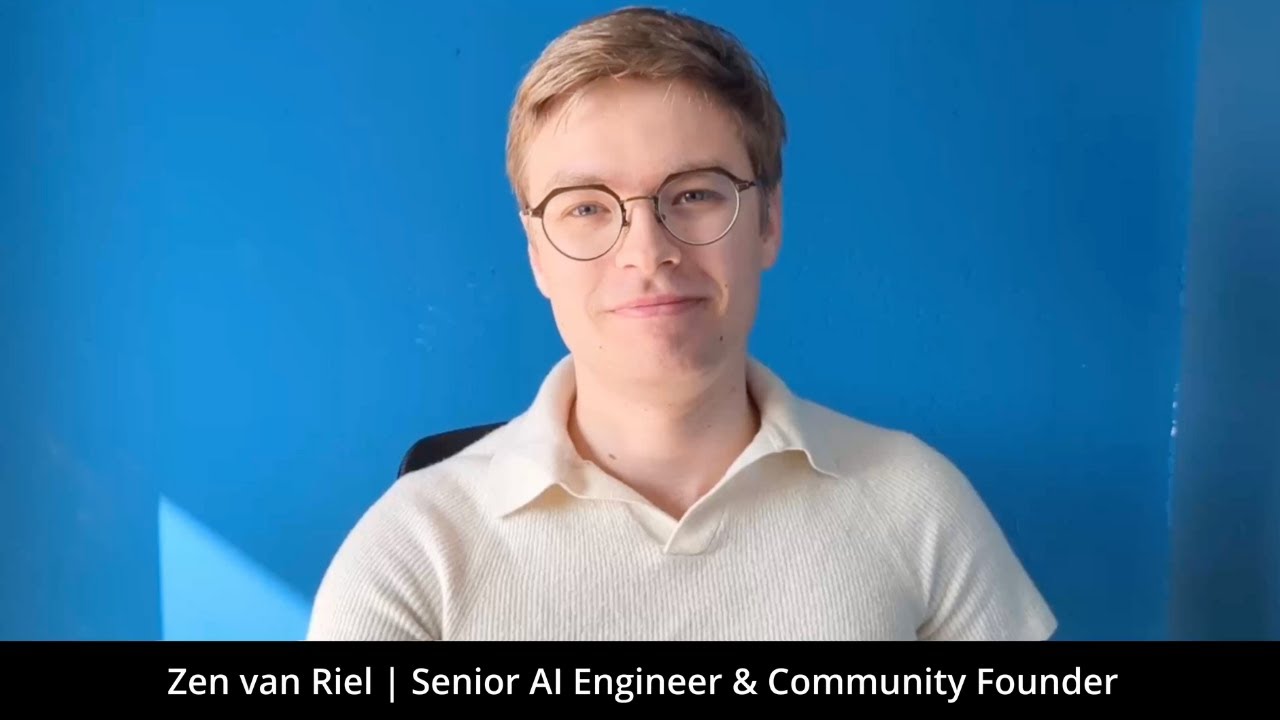
The Ultimate AI Engineer Job Guide For Skills, Salaries and Growth
When I was 20 years old and just starting my journey in tech, I couldn’t have imagined how dramatically my career and income would transform through AI engineering. I went from an uncertain beginner to a senior engineer at big tech with a six-figure salary in just four years. But what’s even more valuable is knowing I’ve built a career that’s actually strengthened by AI advancement rather than threatened by it.
My Personal Compensation Journey
Let me be transparent about my own path: I almost tripled my income since starting as a new grad. This wasn’t due to luck or extraordinary talent – it came from strategically focusing on the skills companies desperately need but can’t find enough people to fill.
I’ve seen firsthand how the demand for AI Engineers has grown approximately 3x in recent years. This growth has created a remarkable compensation landscape:
- In higher-end markets of Europe (like the Netherlands where I’ve worked), median total compensation reaches around $95,000
- In the United States, roles typically start from $100,000, with significant additional compensation through equity
What surprised me most wasn’t just the starting salary – it was how quickly advancement can happen when you demonstrate genuine implementation ability. The ceiling is remarkably high, and the growth potential is what makes this career path so compelling.
The Skills That Actually Advanced My Career
When I reflect on what actually propelled me from junior to senior engineer at big tech so quickly, it wasn’t theoretical knowledge. It was these specific capabilities:
Production Implementation Experience: I focused on learning how to move AI systems from concept to functioning production environments. At big tech, this meant building systems that thousands of users rely on daily.
Business Problem Solving: I developed the ability to translate business requirements into effective AI solutions with measurable ROI. This skill alone dramatically increased my value to employers.
Data Architecture Knowledge: I invested time in understanding how to structure, store, and process the massive amounts of data that power effective AI systems.
Full Development Lifecycle: Rather than specializing in just one part of AI development, I gained experience with the complete process from initial concept to maintenance.
Communication Skills: I learned how to translate complex technical concepts for non-technical stakeholders, which became crucial for advancing projects and my career.
These skills set me apart in job interviews and promotion discussions because relatively few candidates can demonstrate them, despite many claiming AI expertise on their resumes.
My Career Progression Path
My personal journey followed what I now recognize as an accelerated version of the technical specialist track:
At 20, I independently started learning software development and AI while studying full-time. By 21, I joined Microsoft as a junior customer engineer through an internship. At 22, I made the strategic move to become an Azure DevOps engineer to gain more hands-on experience. By 23, I started as a media software engineer at big tech. At 24, I was promoted to senior software engineer.
This progression happened much faster than I initially thought possible, and it’s why I’m so passionate about helping others achieve similar acceleration in their careers.
Why I Believe This Career Has Long-Term Value
Based on my experience implementing AI systems at big tech, I’ve developed strong convictions about the future growth prospects of this field:
Continuous Evolution: As I build new AI capabilities at big tech, I see firsthand how implementation expertise must evolve in parallel, maintaining the value of experienced engineers.
Widening Application: Every month, I encounter new domains seeking specialized AI implementation knowledge, creating ongoing opportunities.
Complexity Barrier: The gap between understanding AI concepts and successfully implementing production systems remains significant – exactly where I’ve positioned my career.
This is why I consider AI engineering skills to be one of the best investments I’ve made in my professional future. While other career paths face potential disruption from AI, those who implement these systems will remain essential.
How I Built My Portfolio (And How You Can Too)
When I was starting out, I focused on creating a portfolio that demonstrated implementation ability through:
Production-Grade Projects: I built complete AI systems that solved real problems, not just tutorial examples.
Business Impact Documentation: I clearly articulated the business value of my implementations, focusing on potential ROI.
Full-Stack Demonstrations: I showcased capabilities across the entire implementation stack to position myself as a complete solution provider.
This approach helped me stand out as someone who could deliver business value through AI, not just discuss theoretical concepts.
If you’re interested in becoming a high paid AI engineer, join the AI Engineering community where we share insights, resources, and support for your learning journey.