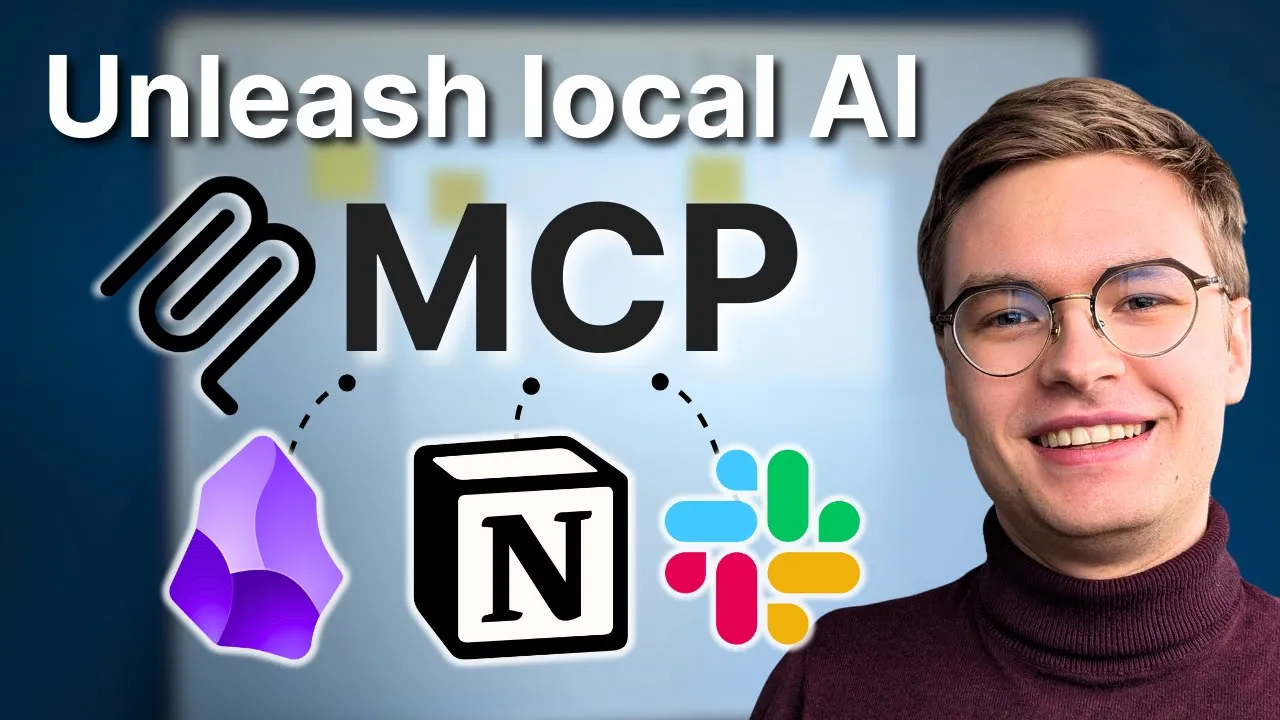
Enhancing Knowledge Management with AI
Personal knowledge management has evolved dramatically in recent years, with tools like Obsidian empowering users to create interconnected knowledge bases. Yet even the most diligent note-takers encounter a persistent challenge: discovering meaningful connections across disparate information sources. This is where artificial intelligence offers transformative potential.
The Knowledge Discovery Gap
Traditional knowledge management relies heavily on manual curation. We create notes, occasionally link related concepts, and attempt to organize information in ways that make sense to our future selves. However, this approach has inherent limitations:
- Our brains naturally categorize information, potentially overlooking non-obvious connections
- The volume of information we collect often exceeds our capacity to manually process it
- Time constraints limit our ability to regularly review and connect existing knowledge
- Our own biases may prevent us from seeing valuable relationships between concepts
These limitations create what we might call the “knowledge discovery gap”—the space between the potential insights hidden in our notes and those we actually discover.
AI as a Connection-Maker
AI excels precisely where humans struggle—rapidly processing large volumes of information and identifying patterns without preconceived notions. When applied to knowledge management, AI can:
- Analyze content across multiple notes simultaneously
- Identify conceptual similarities even when terminology differs
- Suggest connections based on semantic understanding rather than exact keyword matches
- Generate new ideas by combining insights from different domains
The result is a knowledge system that actively participates in making connections rather than passively storing information.
From Storage to Synthesis
The integration of AI with knowledge management systems represents a fundamental shift from information storage to knowledge synthesis. Consider a practical example where AI connects notes on cloud infrastructure with application development documentation:
The AI might identify that certain cloud services provide specialized capabilities for AI applications, suggesting integration patterns you hadn’t considered. These connections transform isolated technical notes into a cohesive framework of related concepts and practical applications.
This synthetic approach creates several benefits:
- Discovery of previously unrecognized relationships between concepts
- Identification of knowledge gaps and areas for further exploration
- Cross-pollination of ideas across different domains of expertise
- Generation of novel approaches to existing problems
Enhancing Learning and Creativity
Perhaps the most valuable aspect of AI-enhanced knowledge management is its impact on learning and creativity. By surfacing unexpected connections, AI challenges our existing mental models and encourages intellectual growth.
This leads to:
- Accelerated learning through conceptual bridging
- Enhanced creativity through unusual juxtapositions
- More comprehensive understanding of complex topics
- Reduced cognitive blind spots and biases
For researchers, writers, and knowledge workers, these benefits translate directly into better outputs and more innovative thinking.
The Future of Knowledge Management
As AI integration with knowledge management systems becomes more sophisticated, we can anticipate even more powerful capabilities:
- Proactive suggestion of relevant resources based on current work
- Automatic organization of information into coherent knowledge structures
- Generation of new content that synthesizes existing knowledge in novel ways
- Identification of emerging patterns across a body of work over time
These advances point toward knowledge management systems that function less like digital filing cabinets and more like collaborative thought partners, actively contributing to our intellectual growth.
Balancing AI Assistance with Human Direction
While AI offers powerful capabilities for knowledge management, the most effective approach combines AI analysis with human direction. The goal isn’t to outsource thinking to AI but to leverage its pattern-recognition capabilities while maintaining human judgment about which connections are truly meaningful.
This balanced approach ensures that AI serves as an extension of human thinking rather than a replacement for it—enhancing our capabilities without diminishing our intellectual agency.
To see exactly how to implement these concepts in practice, watch the full video tutorial on YouTube. I walk through each step in detail and show you the technical aspects not covered in this post. If you’re interested in learning more about AI engineering, join the AI Engineering community where we share insights, resources, and support for your journey. Turn AI from a threat into your biggest career advantage!