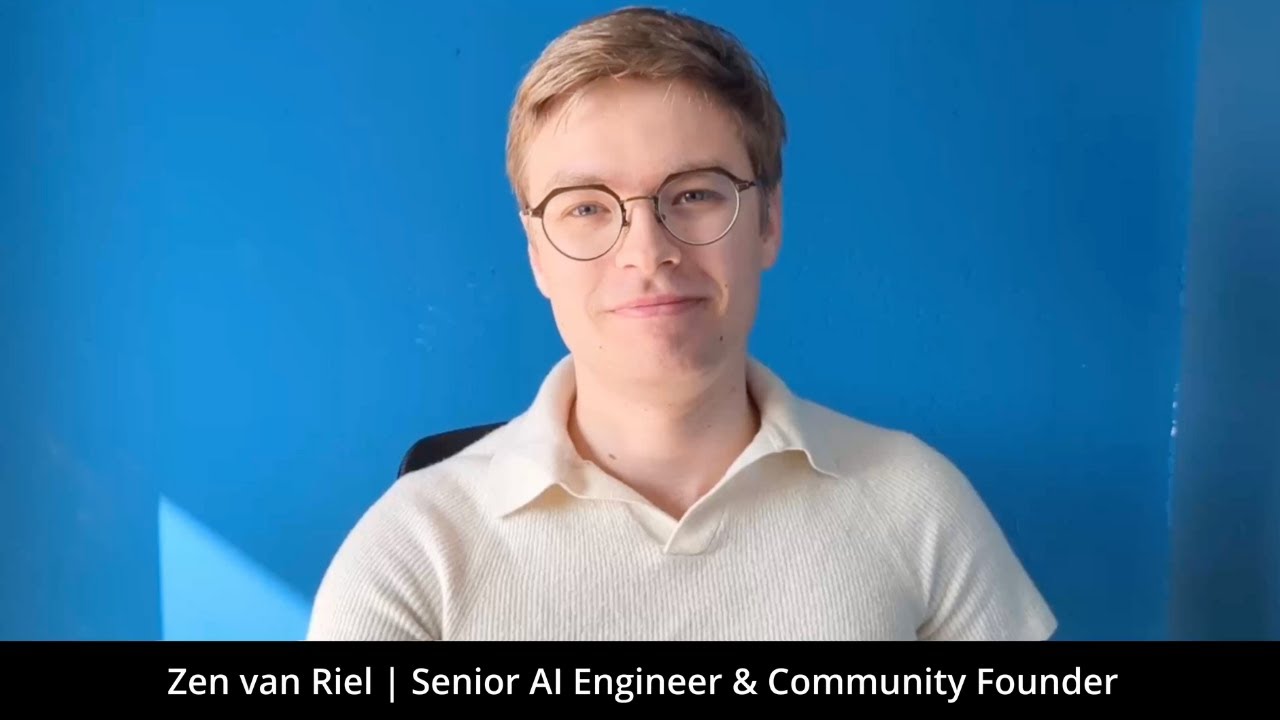
The Half Right AI Revolution
A counterintuitive truth is reshaping the workplace: AI systems that are only moderately accurate but dramatically faster are creating more business value than slower, more precise human approaches. Throughout my experience implementing AI solutions at scale, I’ve observed how this speed-accuracy tradeoff transforms productivity across industries. Organizations increasingly prefer rapid, “good enough” results over slower perfection, shifting value to those who can implement and refine AI systems rather than those who perform tasks that AI can now handle with reasonable accuracy at much greater speed.
The Speed-Accuracy Value Equation
The fundamental calculation driving AI adoption follows a clear pattern that consistently favors significant speed improvements even with accuracy tradeoffs. The traditional approach measures value as accuracy multiplied by units of work divided by time. The AI-enhanced approach changes this equation: even with reduced accuracy, the dramatically increased units of work per time create substantially more overall value. A common result shows that half the accuracy at 10x the speed creates 5x more value – a compelling business case for AI adoption.
This calculation works because most business contexts can accommodate moderate error rates. Error correction costs are often minimal, with mistakes easily identified and fixed quickly. In many situations, being “directionally correct” is sufficient for decision-making, and initial imperfect results can be rapidly improved through feedback. Many business contexts require only 70-80% correctness to deliver value, with human oversight addressing critical accuracy needs.
The resulting productivity gap between AI-enhanced workers and traditional approaches continues to widen. AI-enhanced professionals produce dramatically more output despite occasional imperfections, while traditional workers create higher quality but at vastly slower rates. This competitive impact has market-wide effects, with customers increasingly accepting “good enough” delivered quickly rather than waiting for perfection.
Industry-Specific Implementation Patterns
This speed-accuracy tradeoff manifests differently across various fields. In content creation and marketing, AI dramatically accelerates production processes. Traditional approaches featured meticulously crafted content requiring significant time, while AI-enhanced methods rapidly generate first drafts requiring minor refinement. Despite occasional style inconsistencies and factual errors, the 8-10x faster initial production enables content strategies previously impossible due to production constraints.
Software development cycles compress through similar implementation patterns. AI-generated code with human review and refinement replaces code written entirely by developers. Despite occasional bugs, inefficient sections, and security oversights, the 3-5x faster feature development enables much quicker delivery and market responsiveness.
Data analysis and business intelligence see perhaps the most dramatic acceleration. Automated analysis with human interpretation replaces comprehensive analyst-driven investigation. Even with some missed patterns and occasional misinterpretations, the 5-20x faster initial analysis enables more frequent and diverse insights within acceptable confidence levels, supporting more data-informed decisions across organizations.
Career Impact and Adaptation Strategies
This value shift creates significant professional implications as output standards fundamentally change. Traditional expectations emphasized careful, highly accurate work at standard pace, while emerging standards demand dramatically higher output with reasonable quality. Performance evaluation increasingly considers volume and speed alongside quality, with “good enough” becoming explicitly acceptable in many contexts.
How professionals create value is transforming in parallel. Activities AI can handle adequately are declining in value, while implementing and enhancing AI capabilities grows increasingly important. Critical judgment shifts toward knowing where precision matters versus where speed takes priority. Strategic focus changes from execution to orchestration, with impact measurement evolving to emphasize results over effort.
Rather than striving for error elimination, professionals must effectively manage acceptable error rates. Verification skills become critical for quickly identifying important issues, along with correction efficiency for rapidly addressing significant problems. Calibrating appropriate tolerance thresholds for different scenarios becomes a vital professional skill.
Implementation Skills for Career Advantage
Several specific capabilities help professionals thrive in this environment. AI orchestration expertise – the ability to implement and manage AI systems effectively – creates significant value beyond what AI alone provides. This includes selecting appropriate tools for specific needs, designing effective integration workflows, implementing appropriate validation processes, and continuously enhancing implementation effectiveness.
Judgment and oversight capabilities ensure AI is applied appropriately across different contexts by identifying critical verification points, determining acceptable variance for different situations, calibrating quality-speed tradeoffs appropriately, and evaluating potential consequences of accuracy limitations.
Augmentation workflow design creates effective human-AI collaboration patterns by breaking work into suitable AI and human components, designing smooth transitions between them, implementing continuous improvement mechanisms, and building appropriate verification into workflows to maximize combined effectiveness.
Organizational Value Transformation
This speed-accuracy shift creates strategic implications as market positions shift based on implementation effectiveness. First-mover advantages grow as quicker delivery creates market position benefits. Volume capabilities matter more as capacity to produce at scale becomes critical for competitiveness. Iterative approaches increasingly outperform careful planning as rapid refinement cycles accelerate organizational learning.
Investment patterns evolve in response, with resources shifting from quality to speed investments and implementation focus growing across organizations. Verification systems expand to provide appropriate oversight, while iteration infrastructure develops to support continuous improvement. Organizations increasingly invest in scaling capacity to handle the increased output volume that AI enables.
Risk approaches undergo recalibration with explicitly defined acceptable mistake rates and verification targeting improving to focus resources on critical accuracy needs. Organizations invest in recovery capabilities for quickly addressing significant issues when they occur, while evolving greater transparency about accuracy limitations in their communications.
Implementation Success Strategies
Several approaches consistently deliver value in this environment. Starting with low-risk applications builds implementation experience while managing potential downside by beginning with non-critical processes where errors have limited consequences, focusing on internal applications before external exposure, and targeting high-volume repetitive tasks.
Implementing appropriate verification layers maintains quality while preserving speed advantages by designing oversight based on consequence severity, implementing statistical quality control to check samples rather than all outputs, developing automated exception flags, and establishing performance dashboards that track both speed and accuracy metrics.
Developing hybrid workflows creates effective human-AI collaboration that maximizes combined strengths by assigning tasks based on comparative advantages, designing clear handoffs between AI and human components, implementing feedback loops for continuous improvement, and establishing effective communication patterns.
Conclusion: The “Good Enough” Revolution
The AI transformation isn’t about perfect automation – it’s about dramatically accelerated processes that deliver acceptable quality at unprecedented speed. This fundamental shift challenges traditional approaches that prioritize accuracy over velocity, creating both threats and opportunities for professionals across industries.
Organizations increasingly recognize that moderate accuracy at 10x speed creates more value than high accuracy at standard pace. This recognition is reshaping expectations, performance standards, and competitive dynamics across the economy, favoring those who can effectively implement and enhance AI systems over those who perform tasks that AI can now handle adequately at much greater speed.
Rather than resisting this transformation or focusing solely on quality advantages, successful professionals are developing implementation capabilities that leverage this speed-accuracy reality. By building skills in AI orchestration, appropriate judgment, and effective human-AI collaboration, you can position yourself to thrive in an environment where “good enough, but incredibly fast” increasingly defines competitive advantage.
If you’re interested in learning more about AI engineering, join the AI Engineering community where we share insights, resources, and support for your journey. Turn AI from a threat into your biggest career advantage!