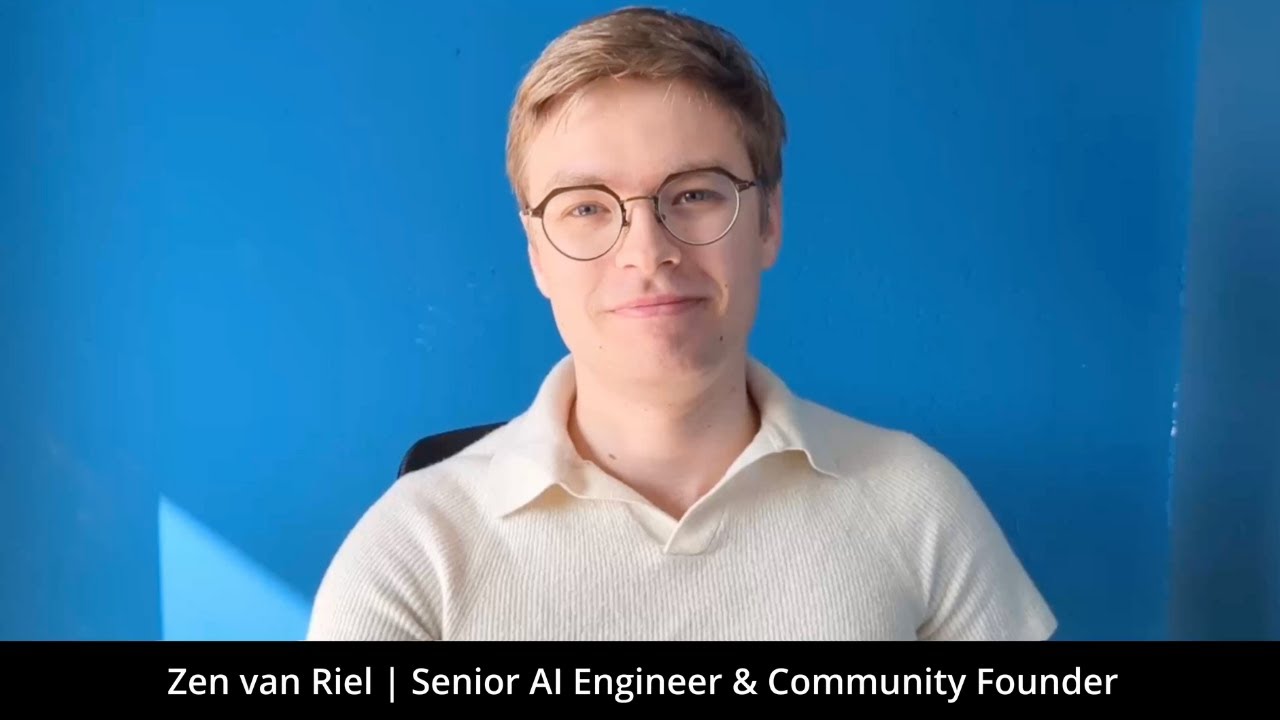
Implementing vs Creating AI Models: Why Companies Need AI Application Engineers Now
When I began my AI journey at 20 years old, I made a decision that completely transformed my career trajectory. Instead of pursuing the theoretical path of creating new AI models, I focused on becoming an AI implementation engineer. This single choice helped me compress a decade-long career path into just four years, going from self-taught developer to Senior Engineer at a big tech company.
The reality is stark but often misunderstood: while AI researchers get the spotlight, it’s AI application engineers who are in overwhelming demand. Let me share why this implementation-focused path is not only more accessible but potentially more valuable for your career.
The Implementation Gap in AI Engineering
When most people think about AI careers, they imagine PhD researchers developing groundbreaking models. But here’s what companies actually need: AI integration developers who can take existing models and implement them to solve real business problems.
During my time at a big tech company, I’ve seen this firsthand. For every research position, there are dozens of openings for engineers who can actually implement AI systems. This implementation gap exists because:
- Most companies don’t need to create new models
- They need engineers who can adapt existing ones
- The real business value comes from successful implementation
This is precisely why I focused my learning on becoming an AI solutions developer rather than a researcher. It allowed me to deliver immediate value while accelerating my career growth.
Building a Production-Ready Skillset
What separated my path from others was my focus on end-to-end implementation. While pursuing my studies, I deliberately developed skills in:
- AI model deployment across different environments
- API integration with existing infrastructure
- Building reliable AI applications that scale
These skills aren’t theoretical – they’re what companies desperately need. When I joined a big tech company at 23, I was valued not for creating new AI algorithms but for my ability to implement existing ones in ways that delivered business value.
The transition from a typical software engineer to AI developer isn’t about mastering complex math – it’s about understanding how to architect AI systems that work reliably in production.
The Career Acceleration Effect
The demand for AI application engineers has created a unique opportunity for career acceleration. In my case, I was able to progress from junior to senior roles in record time because I could demonstrate tangible business impact through implementation.
Companies are willing to pay premium salaries for engineers who can bridge the gap between AI’s potential and actual business results. This implementation focus allowed me to nearly triple my income since starting as a new graduate.
More importantly, AI system integration skills provide career resilience. As AI continues to transform industries, those who can implement these systems will remain in high demand regardless of which specific models dominate the landscape.
Conclusion: Implementation Is Your Competitive Advantage
The most valuable insight from my journey is this: mastering AI implementation is your fastest path to a high-impact, well-compensated career in artificial intelligence. While researchers create the models, it’s implementation engineers who create the value.
By focusing on becoming an AI applications engineer rather than a researcher, you position yourself at the center of where companies are actually investing. This path is more accessible, offers faster career progression, and meets an urgent market need.
Rather than competing with PhDs for limited research positions, consider the abundant opportunities in AI implementation where your impact can be immediate and substantial.
If you’re interested in learning more about AI engineering, join the AI Engineering community where we share insights, resources, and support for your journey. Turn AI from a threat into your biggest career advantage!