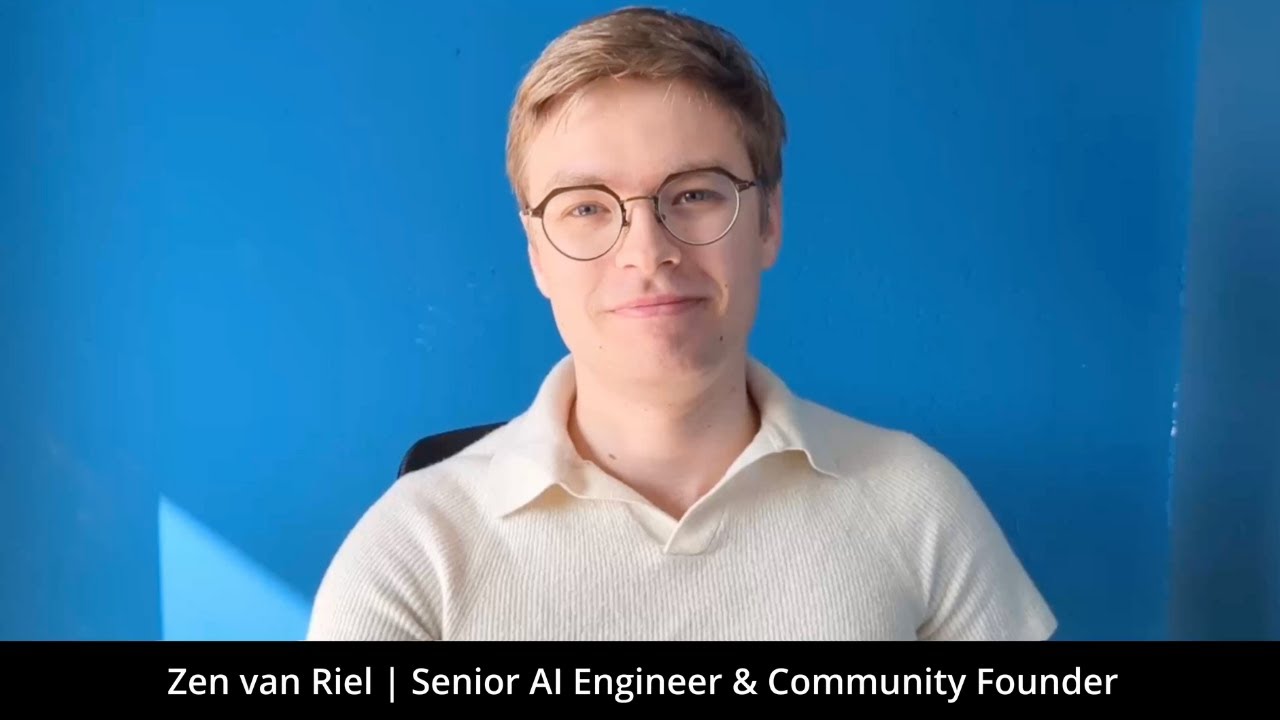
Practical AI Implementation for Operations Managers
As an operations manager, you’re intimately familiar with the promises of efficiency and optimization that come with each new technology wave. Artificial intelligence represents perhaps the most hyped of these advances, with seemingly limitless potential to transform operations. Yet the financial reality is sobering - organizations waste millions on AI initiatives that fail to deliver practical value. Studies consistently show that 80-90% of AI projects in operations never progress beyond pilot phases, resulting in significant resource drain without corresponding benefits. Your role has become essential as the guardian of operational resources against well-intentioned but ultimately impractical AI proposals.
Throughout my experience implementing AI solutions at scale, I’ve observed that successful operational implementations depend not on chasing theoretical capabilities, but on identifying specific, high-value use cases that align with organizational readiness and deliver measurable returns on investment.
The Operations Manager’s AI Implementation Challenge
Operations managers face a unique challenge in the AI landscape. You’re responsible for maintaining efficient, reliable processes while simultaneously identifying opportunities for improvement. AI vendors and consultants present compelling visions of operational transformation, yet translating these concepts into practical implementations requires navigating between hype and genuine opportunity.
This navigation is particularly challenging because operations typically can’t afford the experimental approach common in other departments. Your systems must continue functioning reliably while improvements are implemented, creating a different risk profile than marketing or product development AI initiatives.
Identifying Genuine Operational AI Opportunities
Rather than starting with AI technology, effective operational implementation begins with process understanding. A thorough Process Friction Analysis examines your operations to identify high-value improvement opportunities by documenting process steps that consume disproportionate resources, decision points relying on subjective judgment, repetitive tasks requiring human attention but limited creativity, and information bottlenecks where data exists but isn’t readily accessible.
Next, conduct a Data Readiness Evaluation to assess whether you have the necessary foundation for proposed improvements. Determine if required data is already being captured in accessible systems, evaluate quality and consistency, identify integration requirements across different operational systems, and consider whether historical data reflects current conditions. This helps prioritize opportunities where data foundations already exist, reducing implementation complexity.
Finally, perform an Operational Impact Assessment to quantify potential value. Calculate direct labor savings from automation, estimate error reduction benefits, project capacity increases from accelerated decision-making, and evaluate secondary benefits like improved employee satisfaction. This assessment focuses efforts on opportunities with meaningful operational impact rather than technological novelty.
Practical Steps for Operations Managers Implementing AI
Once you’ve identified promising opportunities, specific implementation approaches can improve your success rate. Start with augmentation rather than replacement by designing initial implementations that enhance human capabilities. Implement AI systems that provide recommendations to operators, create validation frameworks comparing AI and human decisions, maintain manual override capabilities, and collect performance data to guide future development.
Insist on explainable implementations, particularly for operational systems where understanding decision criteria is essential. Require vendors to explain how their systems reach conclusions, implement decision logging, ensure operators can understand and explain system outputs, and develop training that builds understanding of underlying principles. This explainability creates confidence and supports regulatory compliance.
Design for operational realities by involving frontline operators in design and testing phases. Conduct implementation trials during both normal and peak periods, plan for graceful degradation when systems encounter unexpected conditions, and develop clear procedures for when AI systems should be temporarily suspended. These considerations ensure implementations enhance rather than disrupt operations.
Common Operational AI Implementation Pitfalls
Understanding typical challenges helps avoid common missteps. The Perfect Data Fallacy occurs when vendors demonstrate capabilities using idealized scenarios, pilot implementations use carefully curated data, yet real operational data contains exceptions and gaps. Address this by testing with actual operational data early in evaluation.
The Integration Underestimation happens because operational systems rarely exist in isolation. Implementation timelines frequently underestimate integration complexity, performance in isolated testing doesn’t reflect integrated environments, and data flow timing between systems creates synchronization challenges. Mitigate this risk by planning integration from the project outset.
The Change Management Oversight occurs when focus remains solely on technical implementation. Operators need training beyond basic system operation, process documentation requires updating, performance expectations need recalibration, and skepticism about AI capabilities must be addressed directly. Develop comprehensive change management plans alongside technical implementation to address these factors.
Measuring Operational AI Implementation Success
Establish concrete metrics to evaluate implementation outcomes. Track Operational Performance Indicators through process cycle time reduction, error rate changes, resource utilization improvements, and throughput capacity increases. These direct metrics provide clear evidence of implementation value.
Evaluate Implementation Quality Measures through system availability and reliability, exception handling effectiveness, maintenance requirements compared to projections, and operator confidence in system recommendations. These measures help assess whether the implementation provides sustainable operational value.
Connect operational improvements to Business Outcome Alignment by measuring customer satisfaction impacts, cost reductions directly attributable to AI implementation, capacity increases enabling business growth, and compliance improvements from more consistent processes. These connections help communicate implementation value beyond operational departments.
Scaling Successful Operational AI Implementations
Once initial implementations demonstrate value, begin with Documentation and Standardization before scaling. Establish clear documentation of architecture and dependencies, standard operating procedures for monitoring and maintenance, comprehensive training materials, and performance dashboards that track key metrics consistently.
Adopt a Controlled Expansion Approach by applying proven approaches to similar processes, incrementally expanding scope within the same operational area, adapting implementation patterns to adjacent functions, and standardizing components for reuse. This methodical expansion builds on success while managing risk.
Invest in Capability Building by developing internal expertise in successful implementation patterns, creating cross-functional teams combining operational and technical skills, establishing communities of practice to share learning, and building reusable components that accelerate future implementations. These investments transform one-off improvements into systematic operational capability.
Conclusion: Practical Operational Excellence Through AI
As an operations manager, your value in the AI landscape comes not from pursuing theoretical possibilities, but from identifying specific opportunities where current AI capabilities address concrete operational challenges. By focusing on well-defined process friction points, ensuring data readiness, and designing implementations that respect operational realities, you can deliver meaningful improvements while avoiding the pitfalls of AI hype.
Successful operational AI implementation isn’t about revolutionary transformation, but about systematic enhancement of existing processes through carefully selected, well-implemented capabilities. This focused approach delivers tangible value while building the foundation for broader operational innovation.
If you’re interested in learning more about AI engineering, join the AI Engineering community where we share insights, resources, and support for your journey. Turn AI from a threat into your biggest career advantage!