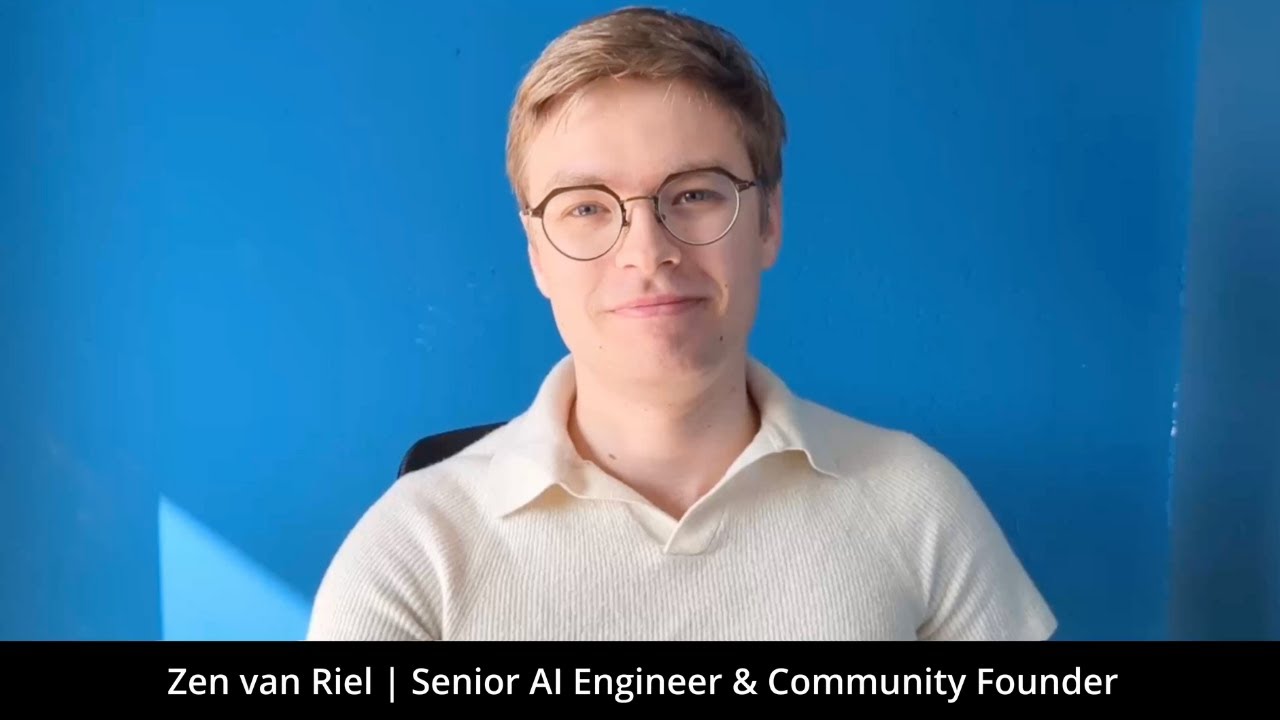
Building AI Talent Strategy
As a Learning & Development leader, you face mounting pressure to develop AI capabilities across your organization. Executive leadership expects talent strategies that will position the company for AI-driven innovation, while employees seek guidance on which skills will secure their future. The financial stakes couldn’t be higher - industry analyses reveal that up to 70% of AI training expenditures fail to produce measurable business outcomes, representing a massive misallocation of development resources.
Yet the gap between AI hype and practical implementation creates significant challenges in determining which capabilities truly matter. Throughout my experience implementing AI solutions at scale, I’ve observed that successful talent strategies focus not on broad theoretical knowledge, but on specific implementation skills that deliver business value and provide measurable return on development investments.
The L&D Leader’s AI Talent Development Challenge
The AI talent landscape presents unique challenges for Learning & Development professionals. Technology vendors promise revolutionary capabilities, while employees worry about obsolescence. Meanwhile, executives expect transformation without necessarily understanding the skills required to achieve it. Organizations frequently invest heavily in theoretical AI knowledge that never translates to practical implementation capability, wasting both financial resources and valuable employee development time.
This navigation is particularly challenging because AI skill needs differ significantly from previous technology waves. The most valuable capabilities often aren’t advanced theoretical knowledge, but practical implementation skills that bridge the gap between AI possibilities and business realities.
Identifying True AI Talent Needs
Effective talent strategies begin with specific organizational needs. The Implementation Gap Analysis assesses your organization’s specific AI implementation challenges by identifying projects that have moved beyond concept but struggle with practical deployment, documenting recurring technical challenges, noting where promising AI concepts fail to translate into working systems, and cataloging situations where AI deliverables don’t meet business stakeholder expectations.
The Capability Spectrum Assessment evaluates your organization’s distribution across different AI capabilities, from research capabilities that generate new concepts to engineering skills that transform concepts into working implementations, integration expertise that connects AI systems with existing processes, and operational knowledge for maintaining and enhancing AI systems. Most organizations over-invest in conceptual knowledge while under-investing in implementation capabilities.
The Role Evolution Framework analyzes how existing roles need to evolve with AI implementation by identifying which positions will need to incorporate AI capabilities, determining which responsibilities might be augmented rather than replaced, assessing where entirely new roles may be needed, and recognizing bridge positions that connect technical and business perspectives.
Practical Talent Strategies for AI Implementation
Develop T-Shaped Implementation Skills by supporting balanced skill profiles that combine deep expertise in specific implementation areas with broader contextual understanding. Focus technical training on practical application rather than theoretical foundations, balance technical capabilities with business domain knowledge, and incorporate project experience alongside formal learning.
Create Implementation-Focused Learning Paths by designing learning journeys that address practical needs. Structure progressive skill development from basic implementation to advanced capabilities, include cross-functional exposure to build comprehensive understanding, incorporate hands-on projects with increasing complexity, and balance vendor-specific capabilities with transferable implementation skills.
Build Communities of Practice by fostering collaborative learning environments where implementation challenges receive collective attention, establishing mentoring relationships between experienced and developing practitioners, developing internal case studies that document successful approaches, and facilitating knowledge exchange across different business units and functions.
Common AI Talent Strategy Pitfalls
The Theoretical Knowledge Overemphasis occurs when training focuses on AI concepts without implementation techniques, learning programs cover algorithms but not engineering practices, employees understand possibilities but lack practical application skills, and certifications verify knowledge but not implementation capabilities. Address this by balancing conceptual understanding with practical application skills in development programs.
The Build-versus-Buy Misalignment happens when organizations invest in developing specialized skills they could more effectively acquire, external hiring targets focus on theoretical rather than implementation expertise, internal development doesn’t prepare employees for actual implementation needs, and career paths don’t reflect the most valuable capabilities. Align talent strategy with realistic assessment of which capabilities can be built versus acquired.
The Role Definition Lag appears when job descriptions fail to incorporate emerging AI implementation responsibilities, career progression doesn’t reflect growing implementation capabilities, performance expectations don’t align with implementation challenges, and compensation models don’t value implementation expertise appropriately. Address these gaps through proactive role redesign based on implementation needs.
Measuring AI Talent Strategy Success
Track Implementation Performance Indicators to see how talent development affects implementation success, including time-to-production improvements for AI initiatives, reduction in external dependencies, increased proportion of concepts reaching operational status, and improved stakeholder satisfaction with implementation outcomes.
Evaluate Capability Development Measures such as increased internal capability to lead end-to-end implementations, reduced reliance on external expertise, growth in mentoring capacity, and expansion of implementation knowledge through internal documentation.
Monitor Talent Sustainability Metrics including engagement levels among implementation specialists, retention rates for key implementation roles, internal mobility into implementation positions, and external recognition of organizational implementation expertise.
Developing AI Implementation Talent at Scale
The Skills Standardization Phase establishes clear competency models for different implementation roles, standardized assessment approaches, structured learning paths with defined progression milestones, and certification frameworks that validate practical implementation skills.
The Targeted Development Approach expands talent initiatives by creating specialized tracks for different implementation specialties, developing role-specific learning journeys, implementing progression frameworks that balance breadth and depth, and establishing clear connections between learning and career advancement.
The Knowledge Capture Investment supports lasting organizational capability by documenting successful implementation approaches, creating internal case studies highlighting implementation lessons, developing scenario-based learning from actual implementation challenges, and building internal knowledge bases that preserve implementation expertise.
Conclusion: Practical Talent Development for AI Success
As an HR or L&D leader, your value in the AI landscape comes not from chasing theoretical skill development, but from building practical implementation capabilities that directly support business outcomes. By focusing on the skills that bridge the gap between AI concepts and operational reality, you can develop talent strategies that deliver genuine value while avoiding the pitfalls of AI hype.
Successful AI talent development isn’t about broad technical training, but about targeted capability building that addresses specific implementation challenges. This focused approach delivers immediate benefits while building the foundation for sustainable competitive advantage through implementation expertise.
If you’re interested in learning more about AI engineering, join the AI Engineering community where we share insights, resources, and support for your journey. Turn AI from a threat into your biggest career advantage!